Reducing the road freight emissions through integrated strategy in the port cities
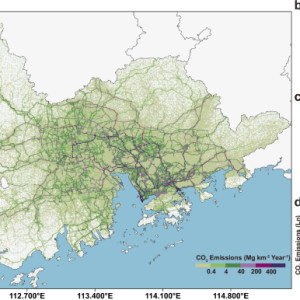
We quantified both the individual and combined mitigation impacts of fleet electrification and road network development on CO2 and other criteria air pollutant emissions from 2016 to 2035 in the Greater Bay Area (GBA) region (Fig. 1). The Integrated Future Emission Projection for Port Cities (IFEPP) framework advances the emission models by enabling the prediction of future emissions and the evaluation of mitigation strategies with nuanced spatial resolution. IFEPP integrates a trajectory-based microscopic vehicle emission model24, traffic flow simulations, and a statistical fleet model (Supplementary Fig. 1), comprehensively incorporating dynamics in freight transportation demands, fleet turnover and electrification, as well as road network structures. A key attempt is the big-data driven future trajectory-level emission modeling and traffic flow simulations. Additionally, historical fleet population data under different emission standards and supply chain demands were utilized to fit the fleet turnover model, predicting future HDT size and composition and to establish a baseline emission scenario.
We conducted scenario analyses across a range of electrification rates under both mixed-energy and ideal renewable energy power grids, coupled with dynamic road network development to assess the mitigation potential and uncertainty ranges of different strategy combinations. This high-resolution emission projection enables the identification of emission hotspots and areas susceptible to emission relocation, providing critical insights for targeted road freight management in port cities and surrounding areas. The IFEPP framework addresses the challenges of integrating diverse elements through a robust data processing pipeline, comprehensive model integration, and dynamic adaptability.
By effectively handling multiple data sources, particularly extensive GPS datasets, it ensures precise trajectory-level emission modeling and traffic flow simulations. The framework can harmonize freight demands, fleet electrification, and road network dynamics to enable high-resolution projections and identify critical emission redistribution patterns, providing actionable insights for effective mitigation strategies.
Fig.1: Spatial distribution of heavy-duty truck emissions from Shenzhen port city to the GBA region in 2016.

a CO2 emissions distribution in 2016 at 0.5 x 0.5 km resolution in GBA regions. b Population distribution near the Shekou port area in Nanshan District, Shenzhen. c Population distribution near the Yantian port area in Yantian District, Shenzhen. d Kernel density estimation plot of population density and HDT CO2 emissions in Shekou and Yantian port areas. *Source data are provided as a Source Data file.
Full size image[1]Data-driven feature identification of road freight emissions
Overall, HDTs from Shenzhen port city emitted ~8.3 (7.5-9.1, 90% confidence interval) x 105 Mg CO2, ~2.3 (2.1-2.5) x 103 Mg carbon monoxide (CO), ~9.2 (8.3-10) x 103 Mg NOx, ~88 (79-97) Mg PM2.5, and ~188 (169-207) Mg hydrocarbons (HC) to the GBA region in 2016 (Supplementary Fig. 2 and Supplementary Table 1). Emissions were predominantly concentrated near the Shekou and Yantian port areas, two of the world's top container port areas (Fig. 1a).
Combined, emissions from these two port areas account for 12% and 13% of the total CO2 and NOx emissions in the GBA, respectively. The limited capacity of existing port infrastructure and urban road networks channels freight traffic into densely populated urban zones. Additionally, the uneven distribution of freight-related facilities across the GBA leads to spatial overlaps between freight corridors and residential areas, intensifying local emissions and increasing human exposure.
Spatial analysis shows that these ports are located within densely populated areas of Shenzhen, housing over 310,000 residents (Supplementary Table 2). In these areas, the annual average NOx emission intensity of 19.7 Mg km-2 year-1 is nearly 100 times greater than the regional average (Fig. 1b, c[2]). Correlation analyses between gridded population density and HDT emissions in Nanshan and Yantian districts, where Shekou and Yantian ports are located, show a significant positive correlation (p < 0.001), highlighting the disproportionate impacts of HDT emissions in densely populated regions (Fig. 1d).
Emission intensity analysis based on high-resolution global emission inventory also reveals that freight emissions are geographically skewed towards major populated port cities worldwide28 (Supplementary Table 3). This further emphasizes the need for targeted emission mitigation strategies in these critical areas.
The frequent regional road freight between ports, industrial zones, and logistics parks has also led to significant CO2 and air pollutant emissions beyond Shenzhen. Notably, 40% of HDTs' total CO2 emissions occurred outside Shenzhen within the GBA. Among the emissions outside Shenzhen, over 60% were emitted in Dongguan, an important stop along the freight corridors.
Based on the 0.5 x 0.5 km resolution emission distribution, we identified seven road links with CO2 emission intensities exceeding 400 Mg km-2 year-1, all of which are major freight corridors connecting the two port areas to industrial and logistics parks in Guangzhou, Foshan, Dongguan, and Zhongshan. The intersection of the emission and population distributions indicates that two million people reside in the grids with high HDT emissions along the corridors (see Supplementary Fig. 3). Based on the IFEPP framework (see Methods), we projected baseline emission trends spanning from 2016 to 2035, considering anticipated changes in the population size and composition of HDTs in line with evolving emission standards.
The HDT population is forecast to expand from around 19,000 in 2016 to 66,000 by 2035 (Supplementary Fig. 4). To address uncertainties in HDT population projections, we applied a technology stock (TS) model (See Supplementary Note 2). The TS model includes truck demand growth rate and retirement rate, which can capture the dynamic evolution of truck demand and decommissioning trends, two key factors influencing uncertainties in scenario results.
Despite higher model error due to data constraints, the predicted HDT population trends from the TS model are in good agreement with those from the autoregressive integrated moving average (ARIMA) model, further supporting the reliability of our projections. The TS model was used for sensitivity analysis by perturbing model parameters, indicating that a 5% uncertainty in truck demand and retirement rates would induce 3.6% and 1.0% uncertainty in the HDT population and corresponding emissions, respectively. The results of sensitivity analysis justify that the uncertainties of the scenario are reasonably considered in our study.
Furthermore, the model's predicted truck growth aligns with the observed trends in China, reinforcing the credibility and real-world relevance of our subsequent scenario analyses. The fleet composition is projected to transition from China IV standards in 2016 to China V from 2017 to 2025, before adopting the stricter China VI thereafter (here, China I-VI standards are the legal requirements in China governing air pollutants released into the atmosphere, which set quantitative limits on the permissible amounts of specific pollutants emitted by designated sources over specific timeframes) (Fig. 2a). Technological advancements to comply with these stricter regulations predominantly involve the adoption of end-of-pipe emission control devices, such as diesel particulate filters (DPFs), selective catalytic reduction (SCR), and exhaust gas recirculation (EGR).
The adoption of stricter emission standards is expected to reduce emissions of PM2.5, NOx, and HC significantly. PM2.5 emissions are projected to decline consistently from 2016 to 2035 due to the incremental tightening of PM2.5 emission standards under China V and China VI, countering the effects of fleet size expansion. For NOx and HC, emissions were expected to peak in 2022 (Fig. 2c).
The upward trend from 2016 to 2022 can be attributed to the expanding truck population, while emission standards for NOx and HC remained consistent between China IV and China V29. A considerable decrease in emissions is anticipated after 2022, following the introduction of China VI--one of the most rigorous HDT emission standards globally30--which is likely to result in a 71% reduction in NOx emissions. In the absence of the China VI standard, NOx emissions could rise to around 2.3 x 104 Mg by 2035. Subsequent discussions on air pollutant emissions will focus primarily on NOx, as it represents the predominant end-of-pipe pollutant from HDTs and is a major target for future regulatory efforts31.
Fig.2: Projection of Shenzhen registered heavy-duty truck emissions in the GBA region from 2016 to 2035.

a Temporal trend of fleet composition in compliance with pre-China I emission standard through China VI emission standard from 2016 to 2035. b, c CO2 and NOx emission trends from 2016 to 2035 and contribution from HDTs in compliance with different emission standards. * Source data are provided as a Source Data file.
Full size image[3]The changes in emission rates applied in the trajectory-based microscopic vehicle emission model are projected based on recommended baseline emission factors from the emission inventory guidebook by the Ministry of Ecology and Environment of the People's Republic of China29 (Supplementary Fig. 5). Comparison with measured on-road NOx emission factors for HDTs from the literature suggests good consistency between measured and projected emission factors for China III to China VI HDTs, in terms of both absolute values and decreasing trends (Supplementary Table 4).
For China I and China II HDTs, the projected emission factors are 36% and 20% lower than the measured values. A sensitivity test using measured on-road emission factors shows that differences in emission factors for China I and China II HDT had minor impacts on NOx emission estimates because old HDTs manufactured in compliance before China III only accounted for 27% of the fleet by 2016 (Supplementary Fig. 6). Contrary to the declining trajectory of other pollutants, emissions of CO2 and CO have consistently risen since 2016, with projections indicating a sustained increase until 2035 at an average annual growth rate of 5.7% (Fig. 2b; Supplementary Table 1).
Relative to current emission levels, this upward trajectory implies an additional 1.5 x 106 Mg of CO2 emissions from HDTs by 2035. This trend correlates with the growing HDT population, since the CO2 and CO emission standards have remained unchanged from China IV onwards. While end-of-pipe control technologies are proficient in curbing PM2.5, NOx, and HC emissions, their efficacy in reducing CO2 and CO emissions is limited because they do not enhance engine fuel efficiency.
Consequently, the emission trajectories of CO2 and CO deviate from those of other pollutants. We performed a sensitivity analysis to assess the extent to which the CO? emission factor needs to be reduced through fuel efficiency improvements to offset the projected increase in HDTs under the China V and VI standards. The findings suggest that CO2 and CO emissions could peak in 2029 if China mandates an aggressive 35% improvement in fuel efficiency no later than 2025, translating to a ~ 30% reduction in CO? emission factors and associated emissions (Supplementary Fig. 7).
Mitigation performance of fleet electrification
Electrification of the fleet stands out as one of the most promising avenues for decarbonizing the transportation sector and synergistically reducing air pollutant emissions3,20,32,33. China leads the electric truck market, which was valued at 2.4 billion US dollars in 2023 and is projected to expand at a compound annual growth rate of 23.26%, reaching 15.6 billion US dollars by 203234,35. This robust growth underscores the promising switch for clean freight technologies in China. Considering that ~27% of HDT emissions are concentrated within 10 km of Shenzhen port, the proximity between ports and logistic parks or industrial zones facilitates the adoption of electric trucks.
In this study, we assessed fleet electrification's impact on reducing road freight emissions up to 2035 by varying heavy-duty electric truck (HDET) growth rates from a conservative 5%34 to an optimistic 35%35,36, in 5% intervals. This sensitivity test determines the necessary growth rate of HDETs to ensure peak CO2 emissions from road freight around 2030. CO2 emissions from electricity generators due to extra electricity demand from HDETs were also considered for two positive future electricity grid scenarios19,37: a sustainable development (SD) scenario assuming 47% renewable energy and an ideal 100% renewable energy scenario.
Before 2025, the HDETs fraction remained low across growth rates, attributed to the limited proportion of HDETs. Post-2025, the difference in HDETs fractions across growth rates widens (Fig. 3a). In the 100% renewable scenario, a CO2 emissions peak can be realized in 2033 or 2030 with a growth rate of 30% or 35% (Fig. 3b).
Relative to the scenario without HDET deployment, CO2 emission reductions by 2035 are estimated at 25% and 48% under the 30% and 35% growth rates. Encouraging HDETs at over a 30% growth rate also supports the declining trend of NOx emissions past the 2030 s (Supplementary Fig. 8). In contrast, CO2 emission will not peak even at a growth rate of 35% under the SD scenario (Fig. 3d).
Under the SD scenario with 47% of the energy generated from renewable energies, HDETs reduce 18.5% of the CO2 emissions (Fig. 3b, c[4]). Although the CO2 emissions from HDETs are relatively lower than those of diesel-powered HDTs, it would take nearly 70% electrification of trucks to contribute to a CO2 peak by 2034 in the SD scenario at a growth rate of 40% (Supplementary Fig. 9). Our findings indicate that the strategy of fleet electrification requires time to curtail the fleet's carbon emissions substantially, even with an ambitious growth rate of 35%.
Sluggish penetration of HDETs or the lack of a low-carbon electricity grid to support HDETs will pose additional challenges to achieving carbon mitigation targets for road freight19.
Fig.3: Mitigation performance of fleet electrification to total CO2 emissions from road freight between 2016 and 2035.

a Anticipated population size of HDETs under different annual growth rates. b, c Total CO2 emissions from HDTs under different annual growth rates of HDETs powered by net-zero emission electricity grids and grids under the SD scenario. d CO2 emission difference from HDETs between the net-zero emission electricity grids and the SD grids scenarios at different annual growth rates. *Source data are provided as a Source Data file.
Full size image[5]Mitigation performance of road connectivity improvements
Driven by the high demand for freight transport between ports and surrounding logistic parks and industrial zones, the total emission and spatial distribution of road freight emissions in port cities depends heavily on road network connectivity. Emission hotspots typically occur in congested nodes or overloaded links38,39,40. To address the rapidly growing freight transport demands, China has been investing heavily in large-scale road network expansion, particularly in key economic regions such as the GBA. By 2023, the country's expressway network surpassed 180,000 km, growing at an average annual growth rate of 5%7, reflecting a strong commitment to enhancing road connectivity and supporting efficient freight distribution.
The planned road network expansion in Shenzhen, aimed at facilitating efficient and reliable freight distribution for the world's busiest container port cluster, provides us with an opportunity to assess the impact of road connectivity improvements on freight emission mitigation. Optimizing transportation routes due to road network development may shorten travel distances, enhance fuel efficiency through improving traffic congestion, and consequently mitigate CO2 and air pollutant emissions41,42. We built a regional road freight traffic flow simulation model (see Methods 3) and simulated alterations in traffic flow and speed resulting from changes in the road network to evaluate the influence of expanding regional road networks on CO2 and NOx emissions for 2016, 2025, 2030, and 2035.
The analysis incorporates the influence of six principal infrastructure initiatives outlined by the Guangdong Provincial Expressway Network Plan 2020-2035, all of which are expected to be operational before 203543. One of the simulated road infrastructures, the Shenzhen-Zhongshan Bridge, connecting Shenzhen port city with a larger region, the western part of the GBA, came into operation in June 2024.
Validation against the observed average speed (56 km h-1) from July 7th to 14th for the bridge shows that the simulated vehicular speed (62 km h-1) was 11% higher44. This comparison demonstrates the satisfactory accuracy of our simulations, considering that traffic flow shortly after opening tends to be higher than its normal volume due to extra tourism travel. Figure 4a delineates the specific simulated area of the six projects.
In a baseline scenario without supplementary road infrastructures, the simulated area's emissions constituted over 30% of the GBA's total emissions of road freight from Shenzhen port city. When contrasted with projections assuming a static road network, route optimization due to road network changes consistently reduced CO2 and NOx emissions across all future years. Increased regional road connectivity over time notably curbed the growth of CO2 emissions within the simulated region.
Specifically, when more direct routes are available, CO2 emissions are projected to increase by only 89%, compared to a 184% rise in the baseline scenario (Fig. 4d). Besides, an additional 11% NOx reduction is attributable to these projects (Fig. 4f). The emission reduction will be most pronounced in 2025 (a 48% reduction in CO2 emissions), coinciding with the start of operation of the Shenzhen-Zhongshan Bridge.
Our simulated statistics indicate that the bridge will reduce detour distances from Shenzhen ports to central logistics parks in Zhongshan by nearly 15 km and substantially improve traffic conditions on the Humen bridges, which serve as vital HDT arteries in the GBA region. However, the additional emission reduction of CO2 following the availability of more direct connecting routes in 2030 and 2035 will fall to 34% and 33%. The diminishing returns of subsequent road network changes emphasize the importance of informed, spatially aware infrastructure planning based on industrial layout and regional freight structure.
Fig.4: Regional road network connectivity improvements and their performance in the reduction of CO2 and NOx emissions from Shenzhen port city HDTs.

a Simulation domain and details of the six road infrastructure enhancement projects. b, c Spatial patterns of CO2 and NOx emissions in 2016, 2025, 2030, and 2035. d, f Comparison between total CO2 and NOx emissions in the simulated area under scenarios with and without road infrastructure projects. e, g Changes in CO2 and NOx emissions contributed by road freight in Guangzhou, Shenzhen, Dongguan, and Zhongshan within the simulated area. The central estimates and uncertainty ranges from (d-g) were derived as p50 and p5-p95 intervals from 10,000 Monte Carlo simulations, with the parameters for the ARIMA model being uniformly distributed and the error margin at 8.8%. *Source data are provided as a Source Data file.
Full size image[6]Spatially, the traffic flow simulations indicate that altered road connectivity may trigger a regional shift in the transshipment activities and cause local enhancements of related emissions according to each city's logistical roles and positions within the restructured network. Cities with improved direct connections--such as Shenzhen and Dongguan--are expected to experience emission relief due to reduced transshipment within urban networks.
Driven by the improved transport connectivity between Shenzhen, Guangzhou, and Zhongshan, these projects are anticipated to redirect emissions towards the southwest of the simulated area (Fig. 4b, c[7]). By 2025, the Shenzhen-Zhongshan Bridge and S78 road are projected to augment Guangzhou's CO2 and NOx emissions by 257% and 181%, respectively (Fig. 4e, g[8]). Cities like Guangzhou and Zhongshan, which have emerged as central transshipment hubs, will witness disproportionately high emission increases due to intensified freight activity.
By 2035, increased connectivity facilitated by the Shenzhu and S0 roads is expected to quadruple Zhongshan's CO2 and NOx emissions compared to scenarios with a static road network. Taking into account fleet expansion, Zhongshan's CO2 emissions in 2035, under the dynamic road network scenario, are projected to be 10 times their 2016 levels (Fig. 4e). Furthermore, Zhongshan is projected to contribute over half of the CO2 and NOx emissions in the simulated region, surpassing Shenzhen as the primary source.
This finding highlights that the increased emissions from the realignment of freight activity cannot be entirely offset by more stringent end-of-pipe emission regulations.
Synergistic performance of integrated strategy
Electrifying HDTs can significantly reduce emissions in the long term, but its immediate impact is limited by low adoption rates and the continued reliance on non-renewable energy sources. Conversely, expanding road networks can lower near-term emissions by reducing detours and alleviating congestion. However, the rapid increase in vehicle numbers and freight demands drives emissions upward, preventing the achievement of peak emission levels.
Therefore, a comprehensive strategy that integrates fleet electrification with strategic road network development is essential. Future emission projections integrating fleet electrification with road network development reveal that improved road connectivity can significantly reduce the CO2 emissions from road freight, as demonstrated in Fig. 5a. In fact, the road connectivity improvement is expected to be more effective than fleet electrification in curbing CO2 and NOx emissions by 2025 and 2030, when the overall electrification rate is still likely to be below 30% (Fig. 5b).
By 2035, however, the electrification of HDTs, supported by a net-zero emission electricity grid, should outperform in NOx emission reduction, while the gains in CO2 emission reduction from road network development should remain more significant. Although road connectivity improvements can effectively lower the growth rate of HDT emissions, they cannot reverse the increasing trend of CO2 emissions driven by the expanding fleet size. Eventually, the emission mitigation potential of fleet electrification is projected to increase rapidly enough to cap CO2 emissions by 2035, assuming a quarter of HDTs are electrified with net-zero emission electricity grids.
Overall, the combination of road connectivity improvement and electrification offers timely CO2 emission reductions while ensuring the achievement of long-term decarbonization goals. Compared to the peak CO2 emission levels from electrification alone with a net-zero emission electricity grid, the peak levels are estimated to be approximately 34% lower when dynamic road networks are considered.
Fig.5: Emission performances of different mitigation strategy scenarios from 2016 to 2035.

a Comparison of CO2 and NOx emissions in the simulated areas under baseline scenario (SC1), individual fleet electrification or road network development (SC2, SC3, and SC4), and coupling strategies (SC5 and SC6). b-d Changes of CO2 emissions between baseline scenario (SC1) and coupling strategy scenario (SC5) in the entire simulated area and Guangzhou and Zhongshan. Changes attributable to fleet electrification and road network development are also displayed. *Source data are provided as a Source Data file.
Full size image[9]The synergy of fleet electrification and road connectivity improvement can further reduce NOx emissions from road freight beyond the baseline scenario, in which China VI HDTs dominate the fleet.
Road accessibility improvement is particularly effective in mitigating near-term NOx emission increases between 2016 and 2025, before the transition from China V to China VI HDTs. When combined with 100% renewable electricity grids, fleet electrification and road network development together are projected to achieve an additional 43% reduction in NOx emissions by 2035. A comparative analysis of investment costs between fleet electrification and road network development as strategies to reduce CO? emissions from HDTs demonstrates that fleet electrification (See Method 4), which includes the acquisition of electric trucks and the installation of charging stations, offers a high return on investment when integrated with a 100% renewable energy grid (Supplementary Note 3, Supplementary Table 5).
The cost is approximately 100US dollars per ton of CO? reduced, slightly below the social cost of carbon reported in the previous study45.
The cost per ton of CO? emission reduction is over five times higher when paired with a mixed-energy power grid due to additional CO? emissions from electricity generation, which limits the overall emission reduction achieved. In contrast, the road network development scenario exhibits investment costs two to three orders of magnitude higher due to the substantial expenses associated with infrastructure construction. Its cost per ton of CO? reduced is also expected to increase over time due to the diminishing benefits of new roads in improving connectivity and reducing emissions in subsequent infrastructure projects.
Notably, the results of the comparison between these two strategies need to be understood in the context of this study. This is because road infrastructure investments serve broader economic and social purposes beyond emissions reduction, such as fostering regional economic development and enhancing connectivity, thereby providing benefits beyond the freight sector. Therefore, to optimize emission mitigation while managing costs, it is imperative to strategically prioritize infrastructure improvements alongside accelerated fleet electrification supported by renewable power grids.
Road network enhancements should focus on alleviating freight congestion and addressing high-emission corridors rather than indiscriminate expansion. This strategic approach ensures substantial emission reductions where most needed, avoids the diminishing returns associated with widespread road construction, and maximizes near-term mitigation benefits for public health. Additionally, expediting the electrification of HDTs, particularly in areas with high freight activity and existing infrastructure, is essential to fully realize emission reduction potential.
Integrating fleet electrification with a robust renewable energy grid ensures the sustainable operation of electric HDTs, thereby enhancing the cost-effectiveness of this strategy. This comprehensive approach guarantees immediate and long-term sustainability in port cities. HDETs can also help to address the regional emission shifts triggered by the newly developed or planned road infrastructures, as evidenced in our road freight traffic flow simulation and emission projection from road network change results.
The adoption of HDETs can counteract the increased emissions in Guangzhou and Zhongshan, induced by the redirected road freight routes through newly constructed and planned road infrastructures connecting these cities with Shenzhen (Fig. 5c, d[10]). In Guangzhou, the promotion of HDETs at an annual growth rate of 30% is expected to neutralize the redirected emissions significantly. In Zhongshan, HDETs are likely to mitigate about a quarter of the emissions surge resulting from these road infrastructure projects.
It is important to note that these analyses do not incorporate the spatial distribution of emissions from electricity generators, and thus, emissions related to HDETs are not considered in the spatial assessment.
References
- ^ Full size image (www.nature.com)
- ^ 1b, c (www.nature.com)
- ^ Full size image (www.nature.com)
- ^ 3b, c (www.nature.com)
- ^ Full size image (www.nature.com)
- ^ Full size image (www.nature.com)
- ^ 4b, c (www.nature.com)
- ^ 4e, g (www.nature.com)
- ^ Full size image (www.nature.com)
- ^ 5c, d (www.nature.com)